- November 28 ~ December 9, 2022
- New Orleans, US
- ML/RL
NeurIPS 2022
Thirty-sixth Conference on Neural Information Processing Systems
Thirty-sixth Conference on Neural Information Processing Systems (NeurIPS 2022) will be a Hybrid Conference with a physical component at the New Orleans Convention Center during the first week, and a virtual component the second week. Along with the conference is a professional exposition focusing on machine learning in practice, a series of tutorials, and topical workshops that provide a less formal setting for the exchange of ideas. We look forward to this year's exciting sponsorship and exhibition opportunities, featuring a variety of ways to connect with participants in person. Sony will exhibit and participate as a Diamond sponsor.
Recruiting information for NeurIPS-2022
We look forward to highly motivated individuals applying to Sony so that we can work together to fill the world with emotion and pioneer the future with dreams and curiosity. Join us and be part of a diverse, innovative, creative, and original team to inspire the world.
For Sony AI positions, please see https://ai.sony/joinus/jobroles/.
*The special job offer for NeurIPS-2022 has closed. Thank you for many applications.
EXPO
- Date & Time
- November 28 (Monday) 08:30-09:30 (PST)
- Venue
- Theater A
- Event Type
- Expo Talk Panel
CHALLENGES & OPPORTUNITIES FOR ETHICAL AI IN PRACTICE
- Bio
- Alice is the head of the AI Ethics Office for Sony Group and the senior research scientist leading AI ethics at Sony AI. In these roles, Alice leads teams of AI ethics researchers and practitioners who work closely with business units to develop more ethical AI solutions. Alice also currently serves as a General Chair for the ACM Conference on Fairness, Accountability, and Transparency, the premier multidisciplinary research conference on these topics.
Alice previously served on the leadership team of the Partnership on AI. As the Head of Fairness, Transparency, and Accountability Research, she led a team of interdisciplinary researchers and a portfolio of multi-stakeholder research initiatives. She also served as a Visiting Scholar at Tsinghua University's Yau Mathematical Sciences Center, where she taught a course on Algorithmic Fairness, Causal Inference, and the Law. Core areas of Alice's research include bridging technical and legal approaches to fairness and privacy, developing methods for detecting and mitigating algorithmic bias, and assessing explainability techniques in deployment.
She has been recognized as one of the 100 Brilliant Women in AI Ethics, and has been quoted in the Wall Street Journal, MIT Tech Review, Fortune, and VentureBeat, among others, for her work on algorithmic bias and transparency, criminal justice risk assessment tools, and AI ethics. She has given guest lectures at the Simons Institute at Berkeley, USC, Harvard, SNU Law School, among other universities. Her research has been published in top machine learning conferences, journals, and law reviews.
Alice is both a lawyer and statistician, with experience developing machine learning models and serving as legal counsel for technology companies. Alice holds a Juris Doctor from Yale Law School, a Master's in Development Economics from Oxford, a Master's in Statistics from Harvard, and a Bachelor's in Economics from Harvard. - Abstract
- In recent years, there has been a growing awareness of the need to consider broader societal impacts when developing and deploying AI models. Research areas like algorithmic fairness, explainability, safety, robustness, and trustworthiness have contributed significantly to our understanding of possible approaches for developing more responsible and ethical AI. Despite these research advances, however, there remain significant challenges to operationalizing such approaches in practice. This talk will discuss technical, legal, and operational challenges that practitioners face when attempting to address issues of bias and lack of transparency in their models. These include tensions between multiple ethical desiderata like fairness and privacy, difficulties of large-scale ethical data collection, and challenges of balancing scalability and bespoke evaluation when designing compliance systems. This talk will also share some of Sony's approaches for addressing these challenges.
Technologies & Business Use case
Technology 01 ToF(Time of Flight) AR
AR technology expresses extended reality enhanced with virtual visual information of onscreen landscapes by utilizing depth information of objects and buildings. It is mainly used in entertainment applications such as for smartphones.
"ToF AR" developed by Sony Semiconductor Solutions Corporation (SSS) can smoothly depict the movements of hands and fingers by using the proprietary AI processing technology jointly developed with R&D Center of Sony Group Corporation. This makes it possible to develop applications that realize the following functionality on Unity : (1) Hand-gesture function, (2) Function to recognize and smoothly depict body movements, (3) Modeling function to create 3D data, (4) Meshing function to build surrounding environments based on depth information, etc.
Technology 02 nnabla-rl: A Flexible Deep Reinforcement Learning Library
Deep reinforcement learning is getting its importance as one of the technology in AI. And we see various application utilize its techniques. However, when applying deep reinforcement learning on a new application, it usually requires to run multiple experiments with different algorithms and settings.
Anticipating such demand, Sony open-sources nnabla-rl, an easy-to-use deep reinforcement learning library which is designed to be used not only for research but also for service & product developments.
More than 30 SoTA deep reinforcement learning algorithms are already implemented in nnabla-rl and the algorithms can be used with only few lines of python code and no expert knowledge of (deep) reinforcement learning is necessary. In nnabla-rl, reinforcement learning algorithms are well modularized, so that users can replace those components easily. This feature will help users to confirm the effect of each algorithm to the performance, and to build a new algorithm without implementing the whole algorithm from scratch.
Technology 03 Bringing video and music to life with deep generative models
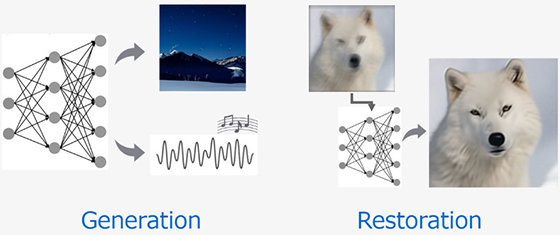
Technologies like deep generative models (DGM) have the potential to transform the lifestyle of consumers and creators. Sony R&D is developing large-scale DGM technologies for content generation and restoration, which we simply call Sony DGM. Current Sony DGM contains two categories: diffusion-based models and stochastic vector quantization technique. We expect Sony DGM to become an integral part of the music, film, and gaming industries in the years to come, and knowing that we at Sony R&D have the unique opportunity to work directly with world-leading entertainment groups within these industries, we want to make the most of this possibility. Demonstration of media generation and restoration available from the https://sony.github.io/creativeai.
Technology 04 Enhancing games with cutting-edge AI to unlock new possibilities for game developers and players.
We are evolving Game-AI beyond rule-based systems by using deep reinforcement learning to train robust and challenging AI agents in gaming ecosystems. This technology enables game developers to design and deliver richer experiences for players. The recent demonstration of Gran Turismo Sophy™, a trained AI that beat world champions in the PlayStation™ game Gran Turismo™ SPORT, embodies the excitement and possibilities that emerge when modern AI is deployed in a rich gaming environment. As AI technology continues to evolve and mature, we believe it will help spark the imagination and creativity of game designers and players alike.
Can an AI outrace the best human Gran Turismo drivers in the world? Meet Gran Turismo Sophy and find out how the teams at Sony AI, Polyphony Digital Inc., and Sony Interactive Entertainment worked together to create this breakthrough technology. Gran Turismo Sophy is a groundbreaking achievement for AI, but there's more: it demonstrates the power of AI to deliver new gaming and entertainment experiences.
Publications
Publication 01 Causality for Temporal Unfairness Evaluation and Mitigation
- Authors
- Aida Rahmattalabi (SonyAI), Alice Xiang (SonyAI)
- Abstract
- Recent interests in causality for fair decision-making systems has been accompanied with great skepticism due to practical and epistemological challenges with applying existing causal fairness approaches. Existing works mainly seek to remove the causal effect of social categories such as race or gender along problematic pathways of an underlying DAG model. However, in practice DAG models are often unknown. Further, a single entity may not be held responsible for the discrimination along an entire causal pathway. Building on the "potential outcomes framework," this paper aims to lay out the necessary conditions for proper application of causal fairness. To this end, we propose a shift from postulating interventions on immutable social categories to their perceptions and highlight two key aspects of interventions that are largely overlooked in the causal fairness literature: timing and nature of manipulations. We argue that such conceptualization is key in evaluating the validity of causal assumptions and conducting sound causal analysis including avoiding post-treatment bias. Additionally, choosing the timing of the intervention properly allows us to conduct fairness analyses at different points in a decision-making process. Our framework also addresses the limitations of fairness metrics that depend on statistical correlations. Specifically, we introduce causal variants of common statistical fairness notions and make a novel observation that under the causal framework there is no fundamental disagreement between different criteria. Finally, we conduct extensive experiments on synthetic and real-world datasets including a case study on police stop and search decisions and demonstrate the efficacy of our framework in evaluating and mitigating unfairness at various decision points.
Publication 02 Men Also Do Laundry: Multi-Attribute Bias Amplification
- Authors
- Dora Zhao (Sony AI), Jerone T. A. Andrews (Sony AI), Alice Xiang (Sony AI)
- Abstract
- As computer vision systems become more widely deployed, there is increasing concern from both the research community and the public that these systems are not only reproducing but amplifying harmful social biases. The phenomenon of bias amplification, which is the focus of this work, refers to models amplifying inherent training set biases at test time. Existing metrics measure bias amplification with respect to single annotated attributes (e.g., computer). However, several visual datasets consist of images with multiple attribute annotations. We show models can learn to exploit correlations with respect to multiple attributes (e.g., {computer, keyboard}), which are not accounted for by current metrics. In addition, \new{we show} current metrics can give the erroneous impression that minimal or no bias amplification has occurred as they involve aggregating over positive and negative values. Further, these metrics lack a clear desired value, making them difficult to interpret. To address these shortcomings, we propose a new metric: Multi-Attribute Bias Amplification. We validate our proposed metric through an analysis of gender bias amplification on the COCO and imSitu datasets. Finally, we benchmark bias mitigation methods using our proposed metric, suggesting possible avenues for future bias mitigation efforts.
Publication 03 A View From Somewhere: Human-Centric Face Representations
- Authors
- Jerone T. A. Andrews (Sony AI), Przemyslaw Joniak (University of Tokyo), Alice Xiang (Sony AI)
- Abstract
- We propose to implicitly learn a set of continuous face-varying dimensions, without ever asking an annotator to explicitly categorize a person. We uncover the dimensions by learning on a novel dataset of 638,180 human judgments of face similarity (FAX). We demonstrate the utility of our learned embedding space for predicting face similarity judgments, collecting continuous face attribute values, and attribute classification. Moreover, using a novel conditional framework, we show that an annotator's demographics influences the importance they place on different attributes when judging similarity, underscoring the need for diverse annotator groups to avoid biases.
Publication 04 A View From Somewhere: Human-Centric Face Representations
- Authors
- Jerone T. A. Andrews (Sony AI), Przemyslaw Joniak (University of Tokyo), Alice Xiang (Sony AI)
- Abstract
- Biases in human-centric computer vision models are often attributed to a lack of sufficient data diversity, with many demographics insufficiently represented. However, auditing datasets for diversity can be difficult, due to an absence of ground-truth labels of relevant features. Few datasets contain self-identified demographic information, inferring demographic information risks introducing additional biases, and collecting and storing data on sensitive attributes can carry legal risks. Moreover, categorical demographic labels do not necessarily capture all the relevant dimensions of human diversity that are important for developing fair and robust models. We propose to implicitly learn a set of continuous face-varying dimensions, without ever asking an annotator to explicitly categorize a person. We uncover the dimensions by learning on a novel dataset of 638,180 human judgments of face similarity (FAX). We demonstrate the utility of our learned embedding space for predicting face similarity judgments, collecting continuous face attribute values, and comparative dataset diversity auditing. Moreover, using a novel conditional framework, we show that an annotator's demographics influences the importance they place on different attributes when judging similarity, underscoring the need for diverse annotator groups to avoid biases.
Publication 05 Value Function Decomposition for Iterative Design of Reinforcement Learning Agents
- Authors
- James MacGlashan, Evan Archer, Alisa Devlic, Takuma Seno, Craig Sherstan, Peter R. Wurman, Peter Stone
- Abstract
- Designing reinforcement learning (RL) agents is typically a difficult process that requires numerous design iterations. Learning can fail for a multitude of reasons and standard RL methods provide too few tools to provide insight into the exact cause. In this paper, we show how to integrate \textit{value decomposition} into a broad class of actor-critic algorithms and use it to assist in the iterative agent-design process. Value decomposition separates a reward function into distinct components and learns value estimates for each. These value estimates provide insight into an agent's learning and decision-making process and enable new training methods to mitigate common problems. As a demonstration, we introduce SAC-D, a variant of soft actor-critic (SAC) adapted for value decomposition. SAC-D maintains similar performance to SAC, while learning a larger set of value predictions. We also introduce decomposition-based tools that exploit this information, including a new reward \textit{influence} metric, which measures each reward component's effect on agent decision-making. Using these tools, we provide several demonstrations of decomposition's use in identifying and addressing problems in the design of both environments and agents. Value decomposition is broadly applicable and easy to incorporate into existing algorithms and workflows, making it a powerful tool in an RL practitioner's toolbox.
Publication 06 Outsourcing Training without Uploading Data via Efficient Collaborative Open-Source Sampling
- Authors
- Junyuan Hong (Sony AI intern), Lingjuan Lyu (Sony AI), Michael Spranger (Sony AI)
- Abstract
- As deep learning blooms with growing demand for computation and data resources, outsourcing model training to a powerful cloud server becomes an attractive alternative to training at a low-power and cost-effective end device. Traditional outsourcing requires uploading device data to the cloud server, which can be infeasible in many real-world applications due to the often sensitive nature of the collected data and the limited communication bandwidth. To tackle these challenges, we propose to leverage widely available open-source data, which is a massive dataset collected from public and heterogeneous sources (e.g., Internet images). We develop a novel strategy called Efficient Collaborative Open-source Sampling (ECOS) to construct a proximal proxy dataset from open-source data for cloud training, in lieu of client data. ECOS probes open-source data on the cloud server to sense the distribution of client data via a communication- and computation-efficient sampling process, which only communicates a few compressed public features and client scalar responses. Extensive empirical studies show that the proposed ECOS improves the quality of automated client labeling, model compression, and label outsourcing when applied in various learning scenarios. Source codes will be released.
Publication 07 Calibrated Federated Adversarial Training with Label Skewness
- Authors
- Chen Chen (Sony AI intern), Yuchen Liu, Xingjun Ma, Lingjuan Lyu (Sony AI)
- Abstract
- Recent studies have shown that, like traditional machine learning, federated learning (FL) is also vulnerable to adversarial attacks. To improve the adversarial robustness of FL, few federated adversarial training (FAT) methods have been proposed to apply adversarial training locally before global aggregation. Although these methods demonstrate promising results on independent identically distributed (IID) data, they suffer from training instability issues on non-IID data with label skewness, resulting in much degraded natural accuracy. This tends to hinder the application of FAT in real-world applications where the label distribution across the clients is often skewed. In this paper, we study the problem of FAT under label skewness, and firstly reveal one root cause of the training instability and natural accuracy degradation issues: skewed labels lead to non-identical class probabilities and heterogeneous local models. We then propose a Calibrated FAT (CalFAT) approach to tackle the instability issue by calibrating the logits adaptively to balance the classes. We show both theoretically and empirically that the optimization of CalFAT leads to homogeneous local models across the clients and better convergence point.
Publication 08 DENSE: Data-Free One-Shot Federated Learning
- Authors
- Jie Zhang (Sony AI intern), Chen Chen (Sony AI intern), Bo Li, Lingjuan Lyu (Sony AI), Shuang Wu, Shouhong Ding, Chunhua Shen, Chao Wu
- Abstract
- One-shot Federated Learning (FL) has recently emerged as a promising approach, which allows the central server to learn a model in a single communication round. Despite the low communication cost, existing one-shot FL methods are mostly impractical or face inherent limitations, e.g. a public dataset is required, clients' models are homogeneous, and additional data/model information need to be uploaded. To overcome these issues, we propose a novel two-stage Data-freE oNe-Shot federated lEarning (DENSE) framework, which trains the global model by a data generation stage and a model distillation stage. DENSE is a practical one-shot FL method that can be applied in reality due to the following advantages: (1) DENSE requires no additional information compared with other methods (except the model parameters) to be transferred between clients and the server; (2) DENSE does not require any auxiliary dataset for training; (3) DENSE considers model heterogeneity in FL, i.e. different clients can have different model architectures. Experiments on a variety of real-world datasets demonstrate the superiority of our method. For example, DENSE outperforms the best baseline method Fed-ADI by 5.08% on CIFAR10 dataset. Our code will soon be available.
Publication 09 CATER: Intellectual Property Protection on Text Generation APIs via Conditional Watermarks
- Authors
- Xuanli He, Qiongkai Xu, Yi Zeng (Sony AI intern), Lingjuan Lyu (Sony AI), Fangzhao Wu, Jiwei Li, Ruoxi Jia
- Abstract
- Previous works have validated that text generation APIs can be stolen through imitation attacks, causing IP violations. In order to protect the IP of text generation APIs, a recent work has introduced a watermarking algorithm and utilized the null-hypothesis test as a post-hoc ownership verification on the imitation models. However, we find that it is possible to detect those watermarks via sufficient statistics of the frequencies of candidate watermarking words. To address this drawback, in this paper, we propose a novel Conditional wATERmarking framework (CATER) for protecting the IP of text generation APIs. An optimization method is proposed to decide the watermarking rules that can minimize the distortion of overall word distributions while maximizing the change of conditional word selections. Theoretically, we prove that it is infeasible for even the savviest attacker (they know how CATER works) to reveal the used watermarks from a large pool of potential word pairs based on statistical inspection. Empirically, we observe that high-order conditions lead to an exponential growth of suspicious (unused) watermarks, making our crafted watermarks more stealthy. In addition, CATER can effectively identify the IP infringement under architectural mismatch and cross-domain imitation attacks, with negligible impairments on the generation quality of victim APIs. We envision our work as a milestone for stealthily protecting the IP of text generation APIs.
Publication 10 Prompt Certified Machine Unlearning with Randomized Gradient Smoothing and Quantization
- Authors
- Zijie Zhang, Xin Zhao, Tianshi Che, Yang Zhou, Lingjuan Lyu (Sony AI)
- Abstract
- The right to be forgotten calls for efficient machine unlearning techniques that make trained machine learning models forget a cohort of data. The combination of training and unlearning operations in traditional machine unlearning methods often leads to the expensive computational cost on large-scale data. This paper presents a prompt certified machine unlearning algorithm, PCMU, which executes one-time operation of simultaneous training and unlearning in advance for a series of machine unlearning requests, without the knowledge of the removed/forgotten data. First, we establish a connection between randomized smoothing for certified robustness on classification and randomized smoothing for certified machine unlearning on gradient quantization. Second, we propose a prompt certified machine unlearning model based on randomized data smoothing and gradient quantization. We theoretically derive the certified radius R regarding the data change before and after data removals and the certified budget of data removals about R. Last but not least, we present another practical framework of randomized gradient smoothing and quantization, due to the dilemma of producing high confidence certificates in the first framework. We theoretically demonstrate the certified radius R' regarding the gradient change, the correlation between two types of certified radii, and the certified budget of data removals about R'.
Publication 11 FairVFL: A Fair Vertical Federated Learning Framework with Contrastive Adversarial Learning
- Authors
- Tao Qi, Fangzhao Wu, Chuhan Wu, Lingjuan Lyu (Sony AI), Tong Xu, Hao Liao, Zhongliang Yang, Yongfeng Huang, Xing Xie
- Abstract
- Vertical federated learning (VFL) is a privacy-preserving machine learning paradigm that can learn models from features distributed on different platforms in a privacy-preserving way. Since in real-world applications the data may contain bias on fairness-sensitive features (e.g., gender), VFL models may inherit bias from training data and become unfair for some user groups. However, existing fair ML methods usually rely on the centralized storage of fairness-sensitive features to achieve model fairness, which are usually inapplicable in federated scenarios. In this paper, we propose a fair vertical federated learning framework (FairVFL), which can improve the fairness of VFL models. The core idea of FairVFL is to learn unified and fair representations of samples based on the decentralized feature fields in a privacy-preserving way. Specifically, each platform with fairness-insensitive features first learns local data representations from local features. Then, these local representations are uploaded to a server and aggregated into a unified representation for the target task. In order to learn fair unified representations, we send them to each platform storing fairness-sensitive features and apply adversarial learning to remove bias from the unified representations inherited from the biased data. Moreover, for protecting user privacy, we further propose a contrastive adversarial learning method to remove privacy information from the unified representations in server before sending them to the platforms keeping fairness-sensitive features. Experiments on two real-world datasets validate that our method can effectively improve model fairness with user privacy well-protected.
Publication 12 BOME! Bilevel Optimization Made Easy: A Simple First-Order Approach
- Authors
- Bo Liu, Mao Ye, Stephen Wright, Peter Stone (Sony AI), and Qiang Liu
- Abstract
- Bilevel optimization (BO) is useful for solving a variety of important machine learning problems including but not limited to hyperparameter optimization, meta-learning, continual learning, and reinforcement learning. Conventional BO methods need to differentiate through the low-level optimization process with implicit differentiation, which requires expensive calculations related to the Hessian matrix. There has been a recent quest for first-order methods for BO, but the methods proposed to date tend to be complicated and impractical for large-scale deep learning applications. In this work, we propose a simple first-order BO algorithm that depends only on first-order gradient information, requires no implicit differentiation, and is practical and efficient for large-scale non-convex functions in deep learning. We provide non-asymptotic convergence analysis of the proposed method to stationary points for non-convex objectives and present empirical results that show its superior practical performance.
Publication 13 (NeurIPS 2022 Workshop on Score-Based Methods) Regularizing Score-based Models with Score Fokker-Planck Equations
- Authors
- Chieh-Hsin Lai, Yuhta Takida, Naoki Murata, Toshimitsu Uesaka, Yuki Mitsufuji (Sony Group Corporation), Stefano Ermon (Stanford University)
- Abstract
- Score-based generative models learn a family of noise-conditional score functions corresponding to the data density perturbed with increasingly large amounts of noise. These pertubed data densities are tied together by the Fokker-Planck equation (FPE), a PDE governing the spatial-temporal evolution of a density undergoing a diffusion process. In this work, we derive a corresponding equation characterizing the noise-conditional scores of the perturbed data densities (i.e., their gradients), termed the score FPE. Surprisingly, despite impressive empirical performance, we observe that scores learned via denoising score matching (DSM) do not satisfy the underlying score FPE. We mathematically analyze two implications of satisfying the score FPE and a potential explanation for why the score FPE is not satisfied in practice. At last, we propose to regularize the DSM objective to enforce satisfaction of the score FPE, and show its effectiveness on synthetic data and MNIST.
Publication 14 MocoSFL: enabling cross-client collaborative self-supervised learning
- Authors
- Jingtao Li (Sony AI), Lingjuan Lyu (Sony AI), Daisuke Iso (Sony AI), Chaitali Chakrabarti, Michael Spranger (Sony AI)
- Abstract
- Existing collaborative self-supervised learning (SSL) schemes are not suitable for cross-client applications because of their expensive computation and large local data requirements. To address these issues, we propose MocoSFL, a collaborative SSL framework based on Split Federated Learning (SFL) and Momentum Contrast (MoCo). In MocoSFL, the large backbone model is split into a small client-side model and a large server-side model, and only the small client-side model is processed locally on the client's local devices. MocoSFL is equipped with three components: (i) vector concatenation which enables the use of small batch size and reduces computation and memory requirements by orders of magnitude; (ii) feature sharing that helps achieve high accuracy regardless of the quality and volume of local data; (iii) frequent synchronization that helps achieve better non-IID performance because of smaller local model divergence. For a 1,000-client case with non-IID data (each client has data from 2 random classes of CIFAR-10), MocoSFL can achieve over 84% accuracy with ResNet-18 model.
Publication 15 Proppo: a Message Passing Framework for Customizable and Composable Learning Algorithms
- Authors
- Paavo Parmas, Takuma Seno (Sony AI)
- Abstract
- While existing automatic differentiation (AD) frameworks allow flexibly composing model architectures, they do not provide the same flexibility for composing learning algorithms---everything has to be implemented in terms of back propagation. To address this gap, we invent Automatic Propagation (AP) software, which generalizes AD, and allows custom and composable construction of complex learning algorithms. The framework allows packaging custom learning algorithms into propagators that automatically implement the necessary computations, and can be reused across different computation graphs. We implement Proppo, a prototype AP software package built on top of the Pytorch AD framework. To demonstrate the utility of Proppo, we use it to implement Monte Carlo gradient estimation techniques, such as reparameterization and likelihood ratio gradients, as well as the total propagation algorithm and Gaussian shaping gradients, which were previously used in model-based reinforcement learning, but do not have any publicly available implementation. Finally, in minimalistic experiments, we show that these methods allow increasing the gradient accuracy by orders of magnitude, particularly when the machine learning system is at the edge of chaos.
Other Conferences
-
- October 23~27, 2022
- Kyoto, Japan
- Robotics
IROS 2022
The 2022 IEEE/RSJ International Conference on Intelligent Robots and Systems (IROS 2022)
View our activities -
- August 8~11, 2022
- Vancouver, Canada
- Computer Vision
SIGGRAPH 2022
The Premier Conference & Exhibition on Computer Graphics & Interactive Techniques
View our activities