- 7 - 14 FEB 2023
- Washington, DC, US
- ML/General AI
AAAI-2023
The 37th AAAI Conference on Artificial Intelligence
AAAI-23 is the Thirty-Seventh AAAI Conference on Artificial Intelligence. The theme of this conference is to create collaborative bridges within and beyond AI. Along with the conference is a professional exposition focusing on machine learning in practice, a series of tutorials, and topical workshops that provide a less formal setting for the exchange of ideas. We look forward to this year’s exciting sponsorship and exhibition opportunities, featuring a variety of ways to connect with participants in person. Sony will exhibit and participate as a Diamond sponsor.
Recruiting information for AAAI-2023
We look forward to highly motivated individuals applying to Sony so that we can work together to fill the world with emotion and pioneer the future with dreams and curiosity. Join us and be part of a diverse, innovative, creative, and original team to inspire the world.
For Sony AI positions, please see https://ai.sony/joinus/jobroles/.
* The special job offer for AAAI-2023 has closed. Thank you for many applications.
Job Fair
- Date & Time
- February 11 (Sat) 12:30 PM - 3:00 PM
- Location
-
Job Fair Theater, in the back of the AAAI Exhibition area
(Hall D of the Walter E. Washington Convention Center)
Sony will be attending the AAAI/ACM SIGAI official Job Fair.
Sony's Global Talent Acquisition Team (HR) will be attending the fair from 12:30 PM to 3:00 PM. Members of HR will also be stationed at the Sony booth in the Exhibition area (Hall D) during the exhibition days (Feb 9th ~ 12th, except during the Job Fair), so please visit us to chat!
We are also holding live online (Zoom) information sessions exclusively for AAAI-23 attendees.
Please register to join us below!
Session #1
- Date & Time
- February 11, 2022 at 6:30 PM (ET)
Session #2
- Date & Time
- February 16, 2022 at 9:00 PM (ET)
We are greatly excited to connect with you at AAAI 2023!
Reference
- Job Fair official site
- https://aaaijobfair.com/
- AAAI-23 Registration
- https://aaai.org/Conferences/AAAI-23/registration/
- Walter E. Washington Convention Center
- https://eventsdc.com/
Workshop Presentation
R2HCAI: The AAAI 2023 Workshop on Representation Learning for Responsible Human-Centric AI
- Date
- February 13, 2023
- Location
- Walter E. Washington Convention Center Washington DC, USA
- Registration
-
Please use the given link to find the details of registration.
Register here.
- Flickr Africa: Examining Geo-Diversity in Large-Scale, Human-Centric Visual Data
(Speakers/Authors)
Keziah Naggita / Julienne LaChance (Sony AI) / Alice Xiang (Sony AI) - A Case Study in Fairness Evaluation: Current Limitations and Challenges for Human Pose Estimation
(Speakers/Authors)
William Thong (Sony AI) / Julienne LaChance (Sony AI) / Shruti Nagpal / Alice Xiang (Sony AI)
Technologies & Business use case
Technology 01 Enhancing games with cutting-edge AI to unlock new possibilities for game developers and players.
We are evolving Game-AI beyond rule-based systems by using deep reinforcement learning to train robust and challenging AI agents in gaming ecosystems. This technology enables game developers to design and deliver richer experiences for players. The recent demonstration of Gran Turismo Sophy™, a trained AI that beat world champions in the PlayStation™ game Gran Turismo™ SPORT, embodies the excitement and possibilities that emerge when modern AI is deployed in a rich gaming environment. As AI technology continues to evolve and mature, we believe it will help spark the imagination and creativity of game designers and players alike.
Can an AI outrace the best human Gran Turismo drivers in the world? Meet Gran Turismo Sophy and find out how the teams at Sony AI, Polyphony Digital Inc., and Sony Interactive Entertainment worked together to create this breakthrough technology. Gran Turismo Sophy is a groundbreaking achievement for AI, but there's more: it demonstrates the power of AI to deliver new gaming and entertainment experiences.
Technology 02 Bringing video and music to life with generative AI
Technologies like generative AI have the potential to transform the lifestyle of consumers and the workflow of professional creators. Sony R&D is developing large-scale generative AI technologies for content generation and restoration, which we simply call Sony generative AI. Current Sony generative AI contains three categories: diffusion-based model, stochastic vector quantization technique, and visual-and-language pre-training.
We expect Sony generative AI to become an integral part of the music, film, and gaming industries in the years to come. Knowing that we at Sony R&D have the unique opportunity to work directly with world-leading entertainment groups within these industries, we want to make the most of this possibility.
Demonstration of media generation and restoration available at https://sony.github.io/creativeai.
Technology 03 Commonsense knowledge acquisition and reasoning for creation with generative AI
Generative systems, such as dialogue agents, story engines, or image generators will change the way humans interact with AI for creative purposes. For these systems to achieve their true potential, though, they must grasp the knowledge that grounds how humans understand the world around them. By understanding the way commonsense knowledge constrains humans’ perception of the world, AI agents will be able to support us in finding novel ways to violate these norms to generate creative outputs. Our work develops resources and algorithms to achieve this goal by teaching machines to (1) acquire and represent the commonsense knowledge underlying the world humans live in, (2) reason over this knowledge to understand situations the same way humans do, and (3) deviate from these knowledgeable and reasonable behaviors to produce sensible creative outputs. Our recent contribution is ComFact, a benchmark for learning and evaluating probabilistic linking models from narratives and dialogues to external knowledge, largely outperforming current heuristic-based linkers:
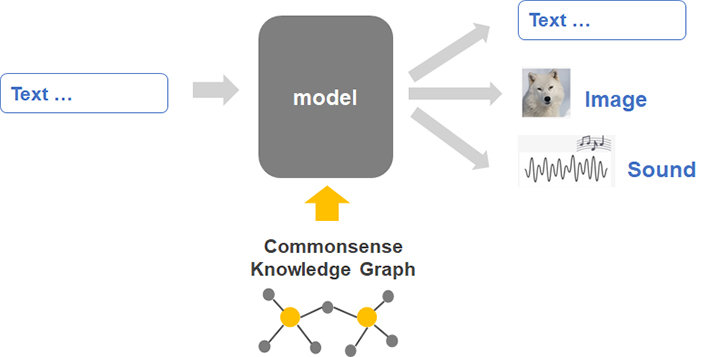
Technology 04 Personalization at Sony
Data-driven personalization is becoming more and more important as Sony provides many DTC (direct-to-consumer) services such as PlayStation Network, Sony LIV, Crunchyroll, and so on. The R&D team has been working on techniques related to personalization in collaboration with the various services with the goal of connecting with 1 billion people.
In our latest research on personalization accepted by AAAI23 (https://arxiv.org/abs/2211.13904) , we focused on off-policy evaluation of models that interact with users. There was no established method for selecting the best estimator to evaluate a model, but we have developed a novel method to select the appropriate estimator adaptively to the situation and established a process by which practitioners can properly perform offline evaluation.
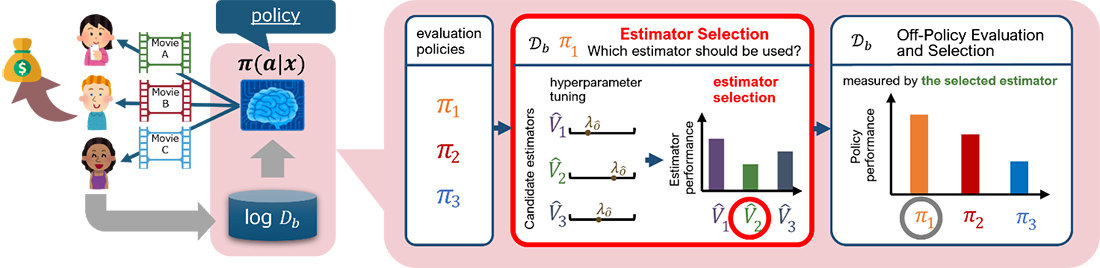
Publications
Publication 01 Metric Residual Networks for Sample Efficient Goal-Conditioned Reinforcement Learning
- Authors
- Bo Liu, Yihao Feng, Qiang Liu, and Peter Stone (Sony AI)
- Abstract
- Goal-conditioned reinforcement learning (GCRL) has a wide range of potential real-world applications, including manipulation and navigation problems in robotics. Especially in such robotics tasks, sample efficiency is of the utmost importance for GCRL since, by default, the agent is only rewarded when it reaches its goal. While several methods have been proposed to improve the sample efficiency of GCRL, one relatively under-studied approach is the design of neural architectures to support sample efficiency. In this work, we introduce a novel neural architecture for GCRL that achieves significantly better sample efficiency than the commonly-used monolithic network architecture. The key insight is that the optimal action-value function Q^*(s, a, g) must satisfy the triangle inequality in a specific sense. Furthermore, we introduce the metric residual network (MRN) that deliberately decomposes the action-value function Q(s,a,g) into the negated summation of a metric plus a residual asymmetric component. MRN provably approximates any optimal action-value function Q^*(s,a,g), thus making it a fitting neural architecture for GCRL. We conduct comprehensive experiments across 12 standard benchmark environments in GCRL. The empirical results demonstrate that MRN uniformly outperforms other state-of-the-art GCRL neural architectures in terms of sample efficiency.
Publication 02 The Perils of Trial-and-Error Reward Design: Misdesign through Overfitting and Invalid Task Specifications
- Authors
- Serena Booth, W. Bradley Knox, Julie Shah, Scott Niekum, Peter Stone (Sony AI), and Alessandro Allievi
- Abstract
- In reinforcement learning (RL), a reward function that aligns exactly with a task's true performance metric is often sparse. For example, a true task metric might encode a reward of 1 upon success and 0 otherwise. These sparse task metrics can be hard to learn from, so in practice they are often replaced with alternative dense reward functions. These dense reward functions are typically designed by experts through an ad hoc process of trial and error. In this process, experts manually search for a reward function that improves performance with respect to the task metric while also enabling an RL algorithm to learn faster. One question this process raises is whether the same reward function is optimal for all algorithms, or, put differently, whether the reward function can be overfit to a particular algorithm. In this paper, we study the consequences of this wide yet unexamined practice of trial-and-error reward design. We first conduct computational experiments that confirm that reward functions can be overfit to learning algorithms and their hyperparameters. To broadly examine ad hoc reward design, we also conduct a controlled observation study which emulates expert practitioners' typical reward design experiences. Here, we similarly find evidence of reward function overfitting. We also find that experts' typical approach to reward design---of adopting a myopic strategy and weighing the relative goodness of each state-action pair---leads to misdesign through invalid task specifications, since RL algorithms use cumulative reward rather than rewards for individual state-action pairs as an optimization target. Code, data: https://github.com/serenabooth/reward-design-perils.
Publication 03 DM2: Decentralized Multi-Agent Reinforcement Learning via Distribution Matching
- Authors
- Caroline Wang, Ishan Durugkar, Elad Liebman, and Peter Stone (Sony AI)
- Abstract
- Current approaches to multi-agent cooperation rely heavily on centralized mechanisms or explicit communication protocols to ensure convergence. This paper studies the problem of distributed multi-agent learning without resorting to centralized components or explicit communication. It examines the use of distribution matching to facilitate the coordination of independent agents. In the proposed scheme, each agent independently minimizes the distribution mismatch to the corresponding component of a target visitation distribution. The theoretical analysis shows that under certain conditions, each agent minimizing its individual distribution mismatch allows the convergence to the joint policy that generated the target distribution. Further, if the target distribution is from a joint policy that optimizes a cooperative task, the optimal policy for a combination of this task reward and the distribution matching reward is the same joint policy. This insight is used to formulate a practical algorithm (DM2), in which each individual agent matches a target distribution derived from concurrently sampled trajectories from a joint expert policy. Experimental validation on the StarCraft domain shows that combining (1) a task reward, and (2) a distribution matching reward for expert demonstrations for the same task, allows agents to outperform a naive distributed baseline. Additional experiments probe the conditions under which expert demonstrations need to be sampled to obtain the learning benefits.
Publication 04 Delving into the Adversarial Robustness of Federated Learning
- Authors
- Jie Zhang (Sony AI), Bo Li, Chen Chen (Sony AI), Lingjuan Lyu (Sony AI), Shuang Wu, Shouhong Ding, Chao Wu.
- Abstract
- In Federated Learning (FL), models are as fragile as centrally trained models against adversarial examples. However, the adversarial robustness of federated learning remains largely unexplored. This paper casts light on the challenge of adversarial robustness of federated learning. To facilitate a better understanding of the adversarial vulnerability of the existing FL methods, we conduct comprehensive robustness evaluations on various attacks and adversarial training methods. Moreover, we reveal the negative impacts induced by directly adopting adversarial training in FL, which seriously hurts the test accuracy, especially in non-IID settings. In this work, we propose a novel algorithm called Decision Boundary based Federated Adversarial Training (DBFAT), which consists of two components (local re-weighting and global regularization) to improve both accuracy and robustness of FL systems. Extensive experiments on multiple datasets demonstrate that DBFAT consistently outperforms other baselines under both IID and non-IID settings.
Publication 05 Defending Against Backdoor Attacks in Natural Language Generation
- Authors
- Xiaofei Sun, Xiaoya Li, Yuxian Meng, Xiang Ao, Lingjuan Lyu (Sony AI), Jiwei Li, Tianwei Zhang
- Abstract
- The frustratingly fragile nature of neural network models make current natural language generation (NLG) systems prone to backdoor attacks and generate malicious sequences that could be sexist or offensive. Unfortunately, little effort has been invested to how backdoor attacks can affect current NLG models and how to defend against these attacks. In this work, by giving a formal definition of backdoor attack and defense, we investigate this problem on two important NLG tasks, machine translation and dialog generation. Tailored to the inherent nature of NLG models (e.g., producing a sequence of coherent words given contexts), we design defending strategies against attacks.
We find that testing the backward probability of generating sources given targets yields effective defense performance against all different types of attacks, and is able to handle the {\it one-to-many} issue in many NLG tasks such as dialog generation. We hope that this work can raise the awareness of backdoor risks concealed in deep NLG systems and inspire more future work (both attack and defense) towards this direction.
Publication 06 Policy-Adaptive Estimator Selection for Off-Policy Evaluation
- Authors
- Takuma Udagawa (Sony), Haruka Kiyohara (Tokyo Institute of Technology), Yusuke Narita (Yale University), Yuta Saito (Cornell University), Kei Tateno (Sony)
- Abstract
- Off-policy evaluation (OPE) aims to accurately evaluate the performance of counterfactual policies using only offline logged data. Although many estimators have been developed, there is no single estimator that dominates the others, because the estimators' accuracy can vary greatly depending on a given OPE task such as the evaluation policy, number of actions, and noise level. Thus, the data-driven estimator selection problem is becoming increasingly important and can have a significant impact on the accuracy of OPE. However, identifying the most accurate estimator using only the logged data is quite challenging because the ground-truth estimation accuracy of estimators is generally unavailable. This paper studies this challenging problem of estimator selection for OPE for the first time. In particular, we enable an estimator selection that is adaptive to a given OPE task, by appropriately subsampling available logged data and constructing pseudo policies useful for the underlying estimator selection task. Comprehensive experiments on both synthetic and real-world company data demonstrate that the proposed procedure substantially improves the estimator selection compared to a non-adaptive heuristic.
Publication 07 Novel Motion Patterns Matter for Practical Skeleton-based Action Recognition
- Authors
- Mengyuan Liu (Sun Yat-sen University)*; Fanyang Meng (Peng Cheng Laboratory); Chen Chen (University of Central Florida); Songtao Wu (Sony R&D Center China)
- Abstract
- Most skeleton-based action recognition methods assume that the same type of action samples in the training set and the test set share similar motion patterns. However, action samples in real scenarios usually contain novel motion patterns which are not involved in the training set. As it is laborious to collect sufficient training samples to enumerate various types of novel motion patterns, this paper presents a practical skeleton-based action recognition task where the training set contains common motion patterns of action samples and the test set contains action samples that suffer from novel motion patterns. For this task, we present a Mask Graph Convolutional Network (Mask-GCN) to focus on learning actionspecific skeleton joints that mainly convey action information meanwhile masking action-agnostic skeleton joints that convey rare action information and suffer more from novel motion patterns. Specifically, we design a policy network to learn layer-wise body masks to construct masked adjacency matrices, which guide a GCN-based backbone to learn stable yet informative action features from dynamic graph structure. Extensive experiments on our newly collected dataset verify that Mask-GCN outperforms most GCN-based methods when testing with various novel motion patterns.
Publication 08 : Workshop on Knowledge Augmented Methods for NLP (KnowledgeNLP-AAAI'23) ComFact: A Benchmark for Contextualized Commonsense Knowledge Linking
- Authors
- Silin Gao (EPFL), Jena Hwang (AI2), Saya Kanno (Sony), Hiromi Wakaki (Sony), Yuki Mitsufuji (Sony), Antoine Bosselut (EPFL)
- Abstract
- Understanding rich narratives, such as dialogues and stories, often requires natural language processing systems to access relevant knowledge from commonsense knowledge graphs. However, these systems typically retrieve facts from KGs using simple heuristics that disregard the complex challenges of identifying situationally-relevant commonsense knowledge (e.g., contextualization, implicitness, ambiguity). In this work, we propose the new task of commonsense fact linking, where models are given contexts and trained to identify situationally-relevant commonsense knowledge from KGs. Our novel benchmark, ComFact, contains ~293k in-context relevance annotations for commonsense triplets across four stylistically diverse dialogue and storytelling datasets. Experimental results confirm that heuristic fact linking approaches are imprecise knowledge extractors. Learned fact linking models demonstrate across-the-board performance improvements (~34.6% F1) over these heuristics. Furthermore, improved knowledge retrieval yielded average downstream improvements of 9.8% for a dialogue response generation task. However, fact linking models still significantly underperform humans, suggesting our benchmark is a promising testbed for research in commonsense augmentation of NLP systems.
Other Conferences
-
- November 28 ~ December 9, 2022
- New Orleans, US
- ML/RL
NeurIPS 2022
Thirty-sixth Conference on Neural Information Processing Systems
View our activities -
- October 23~27, 2022
- Kyoto, Japan
- Robotics
IROS 2022
The 2022 IEEE/RSJ International Conference on Intelligent Robots and Systems (IROS 2022)
View our activities