- June 4 ~ 10, 2023
- Rhodes island, Greece
- Signal Processing
ICASSP 2023
2023 IEEE International Conference on Acoustics, Speech, and Signal Processing (ICASSP 2023)
The International Conference on Acoustics, Speech, & Signal Processing (ICASSP), is the IEEE Signal Processing Society’s flagship conference on signal processing and its applications. This 48th edition of ICASSP will be held in the Greek island of Rhodes. ICASSP’s main theme this year will be “Signal Processing in the Artificial Intelligence era,” promoting the creative synergy between signal processing and machine learning. We look forward to this year's exciting sponsorship and exhibition opportunities, featuring a variety of ways to connect with participants in person. Sony will exhibit and participate as a Platinum sponsor.
Recruiting information for ICASSP-2023
We look forward to working with highly motivated individuals to fill the world with emotion and to pioneer future innovation through dreams and curiosity. With us, you will be welcomed onto diverse, innovative, and creative teams set out to inspire the world.
If interested, please see our full-time and internship roles listed specifically for ICASSP attendees below and consider joining us at the official ICASSP Student Job Fair and Luncheon and/or in-person or on Zoom for our hybrid information session about applying to and working at Sony!
Student Job Fair and Luncheon
Thursday, June 8th 2023 from 12:00 - 15:00 (EEST)
Hybrid Sony Information Session
Friday, June 9th 2023 from 13:15 - 13:45 (EEST)
Register:
https://us02web.zoom.us/webinar/register/WN_F6KQ0jz6TCOu37f5BwyfTQ
For those interested in Japan-based full-time and internship opportunities, please note the following points and benefits:
Japanese language skills are NOT required, as your work will be conducted in English.
Regarding Japan-based internships, please note that they are paid, and that we additionally cover round trip flights, visa expenses, commuting expenses, and accommodation expenses as part of our support package.
Regarding Japan-based full-time roles, in addition to your compensation and benefits package, we cover your flight to Japan, shipment of your belongings to Japan, visa expenses, commuting expenses, and more!
Application Deadline:
Friday, June 16th at 10:00 AM (JST)
*NOTE: Please indicate `ICASSP` for the question that asks ` How did you hear about this position?` in your application, as those who apply through the links below will received expedited application processing.
For Sony AI positions, please see https://ai.sony/joinus/jobroles/.
Contributions to Research Challenges
Challenges 01 No.1 in Task 3 of DCASE 2021
Sony has won the first place in Task 3 of DCASE 2021, the world‘s largest international competition for the detection and classification of acoustic scenes and events. The goal of Task 3 “Sound Event Localization and Detection with Directional Interference” is to recognize individual sound events of specific classes by detecting their temporal activity and estimating their location in the presence of spatial ambient noise and interfering directional events that do not belong to the target classes.
Our system is based on the activity-coupled Cartesian direction of arrival (ACCDOA) representation that enables us to solve a SELD task with a single target. Using the ACCDOA-based system with RD3Net as the backbone, we realize model ensembles. We further propose impulse response simulation (IRS), which generates simulated multi-channel signals by convolving simulated room impulse responses (RIRs) with source signals extracted from the original dataset.
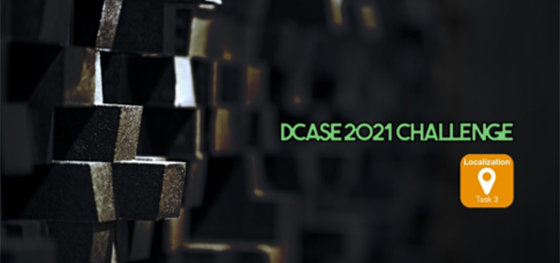
Technical Report
ACCDOA
Kazuki Shimada, Yuichiro Koyama, Naoya Takahashi, Shusuke Takahashi, and Yuki Mitsufuji, “ACCDOA: Activity-coupled cartesian direction of arrival representation for sound event localization and detection,” in Proc. of IEEE ICASSP, 2021.
RD3Net
Naoya Takahashi and Yuki Mitsufuji, “Densely connected multidilated convolutional networks for dense prediction tasks,” in Proc. of IEEE CVPR, 2021.
IRS
Yuichiro Koyama, Kazuhide Shigemi, Masafumi Takahashi, Kazuki Shimada, Naoya Takahashi, Emiru Tsunoo, Shusuke Takahashi, Yuki Mitsufuji, “Spatial data augmentation with simulated room impulse responses for sound event localization and detection, ” in Proc. of IEEE ICASSP, 2022.
Challenges 02 Organizer of Task 3 in DCASE 2023
Continuing from DCASE 2022, Sony has been organizing Task 3 of the DCASE 2023 “Sound Event Localization and Detection Evaluated in Real Spatial Sound Scenes” together with the Audio Research Group (ARG) of Tampere University, where the submitted SELD systems will be evaluated on recordings from real sound scenes. In this challenge there are two tracks that the participants can follow: the audio-only track and the audiovisual track.
For this task, we created Sony-TAu Realistic Spatial Soundscapes 2023 (STARSS23), a new dataset which consists of multichannel recordings and 360° video recordings of real scenes in various room environments along with annotations for spatial and temporal occurrences of target sound events.
Our innovative training scheme called Multi-ACCDOA is used in baseline systems for Task 3 both on audio-only track and the audiovisual track. Implementations are publicly available on GitHub (audio-only track, audiovisual track)
Multi-ACCDOA:
Kazuki Shimada, Yuichiro Koyama, Shusuke Takahashi, Naoya Takahashi, Emiru Tsunoo, Yuki Mitsufuji, “Multi-ACCDOA: Localizing and detecting overlapping sounds from the same class with auxiliary duplicating permutation invariant training,” in Proc. of IEEE ICASSP, 2022.
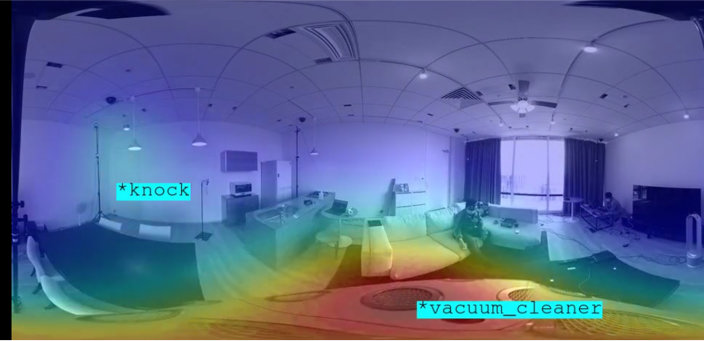
Challenges 03 Sound Demixing Challenge 2023
AICrowd Competition – We organized an online competition for both the research community and industry players together with other two companies, Moises and Mitsubishi Electric Research Labs. In this challenge participants are asked to submit source separation systems in two tracks: the Music Demixing Track (for separating a song into individual musical instruments) and Cinematic Demixing Track (for separating the sound of a movie into dialogue, music and sound effects). In order to evaluate the submitted systems, test datasets made of music tracks of Sony Music Entertainment (Japan) and movies of Sony Pictures Entertainment were used: these were not accessible to the participants and ensured a fair evaluation of the system. The competition was well received by the community, getting in total 2761 submissions from 68 teams around the world.

Challenges 04 Spoken Language Understanding Challenge
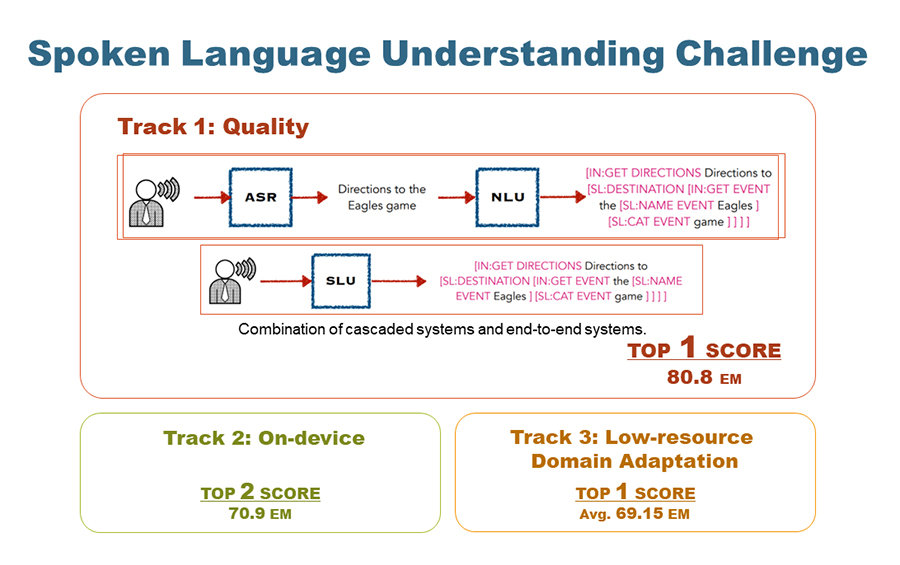
Sony participated with Carnegie Mellon University to Spoken Language Understanding Challenge held at ICASSP 2023. The challenge solves semantic parsing, which is the task of converting a user’s request speech into a structured format for executing tasks. Sony and CMU developed systems for three tracks: Track 1 – quality aiming the highest accuracy (exact match), Track 2 – on-device under 15 M parameters, and Track 3 – low-resource domain adaptation. We proudly achieved first-place at Track 1 with our ROVER model ensemble of cascaded and end-to-end systems, which marked state-of-the-art 80.8% exact match. We also achieved second-place at Track 2 and first-place at Track 3. The reports are presented at the ICASSP conference.
Technologies & Business Use case
Technology 01 Deep Generative Modeling
Technologies like deep generative models (DGM) have the potential to transform the lifestyle of consumers and creators. Sony R&D is developing large-scale DGM technologies for content generation and restoration, which we simply call Sony DGM. We expect Sony DGM to become an integral part of the music, film, and gaming industries in the years to come, and knowing that we at Sony R&D have the unique opportunity to work directly with world-leading entertainment groups within these industries, we want to make the most of this possibility.
Current Sony DGM contains two categories: diffusion-based models and stochastic vector quantization technique. We will briefly introduce our current work below. Demonstration of image generation and media restoration are available from the [Link].
GibbsDDRM
Accepted at ICML 2023 as oral
TL;DR: Solving blind linear inverse problems by utilizing the pre-trained diffusion models in a Gibbs sampling manner.
Pre-trained diffusion models have been successfully used as priors in a variety of linear inverse problems, where the goal is to reconstruct a signal given a noisy linear measurement. However, existing approaches require knowledge of the linear operator. In this paper, we propose GibbsDDRM, an extension of the Denoising Diffusion Restoration Models (DDRM) to the blind setting where the linear measurement operator is unknown. It constructs the joint distribution of data, measurements, and linear operator using a pre-trained diffusion model as the data prior, and solves the problem by posterior sampling using an efficient variant of a Gibbs sampler. The proposed method is problem-agnostic, meaning that a pre-trained diffusion model can be applied to various inverse problems without fine-tuning. Experimentally, it achieves high performance in both blind image deblurring and vocal dereverberation(*) tasks, despite using simple generic priors for the underlying linear operators. This technology is expected to be utilized in content editing in music and film production fields.
(*) We have confirmed that Gibbs DDRM improves the performance of DiffDereverb, which is presented in our ICASSP2023 paper titled "Unsupervised vocal dereverberation with diffusion-based generative models", thanks to the novel sampling scheme.
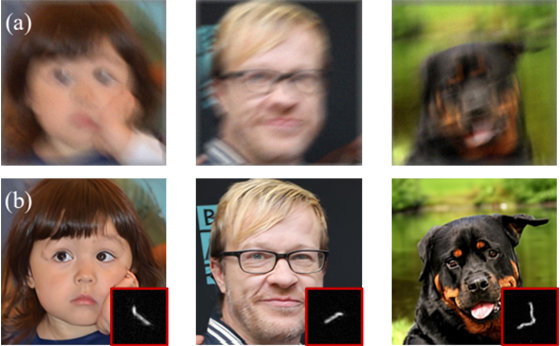
FP-Diffusion
Accepted at ICML 2023
TL;DR: Improving density estimation of diffusion models by regularizing with the underlying equation describing the temporal evolution of scores, theoretically supported.
Diffusion models learn a family of noise-conditional score functions corresponding to the data density perturbed with increasingly large amounts of noise. These perturbed data densities are tied together by the Fokker-Planck equation (FPE), a partial differential equation (PDE) governing the spatial-temporal evolution of a density undergoing a diffusion process. In this work, we derive a corresponding equation, called the score FPE that characterizes the noise-conditional scores of the perturbed data densities (i.e., their gradients). Surprisingly, despite impressive empirical performance, we observe that scores learned via denoising score matching (DSM) do not satisfy the underlying score FPE. We prove that satisfying the FPE is desirable as it improves the likelihood and the degree of conservativity. Hence, we propose to regularize the DSM objective to enforce satisfaction of the score FPE, and we show the effectiveness of this approach across various datasets.
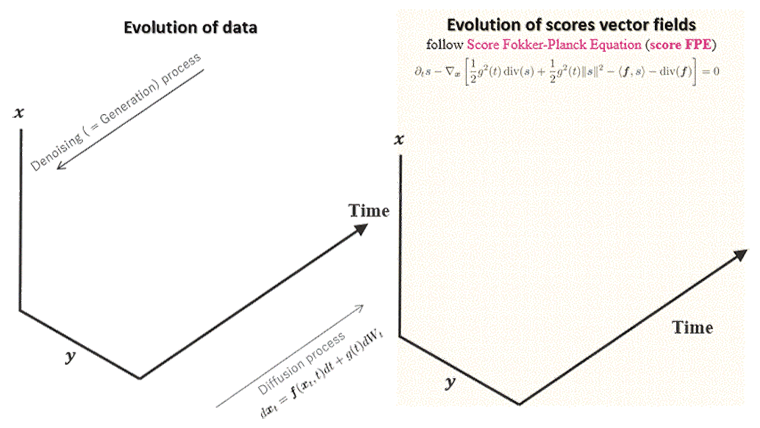
SQ-VAE
Presented at ICML 2022
TL;DR: Training vector quantization efficiently and stably with variational Bayes framework.
One noted issue of vector-quantized variational autoencoder (VQ-VAE) is that the learned discrete representation uses only a fraction of the full capacity of the codebook, also known as codebook collapse. We hypothesize that the training scheme of VQ-VAE, which involves some carefully designed heuristics, underlies this issue. In this paper, we propose a new training scheme that extends the standard VAE via novel stochastic dequantization and quantization, called stochastically quantized variational autoencoder (SQ-VAE). In SQ-VAE, we observe a trend that the quantization is stochastic at the initial stage of the training but gradually converges toward a deterministic quantization, which we call self-annealing. Our experiments show that SQ-VAE improves codebook utilization without using common heuristics. Furthermore, we empirically show that SQ-VAE is superior to VAE and VQ-VAE in vision- and speech-related tasks.
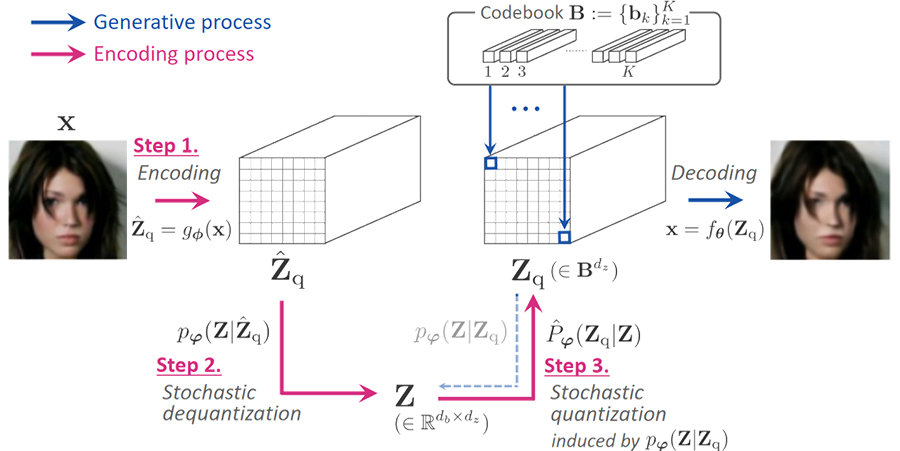
Technology 02 Emotional Voice Synthesis
Voice synthesis has a potential to promote efficiency of creating entertainment contents, such as game, movie and animation. However, this requires not only product-quality synthesis, but also aspects of speaker variations, characteristic uniqueness and acting for the entertainment purpose. Many research organizations among the global Sony family collaborate with each other to contribute to this emotional voice synthesis project. Sony Europe recently developed AutoTTS; a high-quality end-to-end synthesis method with new differentiable duration modelling. Sony Research India realizes emotional voice conversion for speakers whose emotional data was not seen in the training data. Both methods are presented in ICASSP 2023. Our voice synthesis system is recently used in an interactive attraction at the Tokyo Matrix.
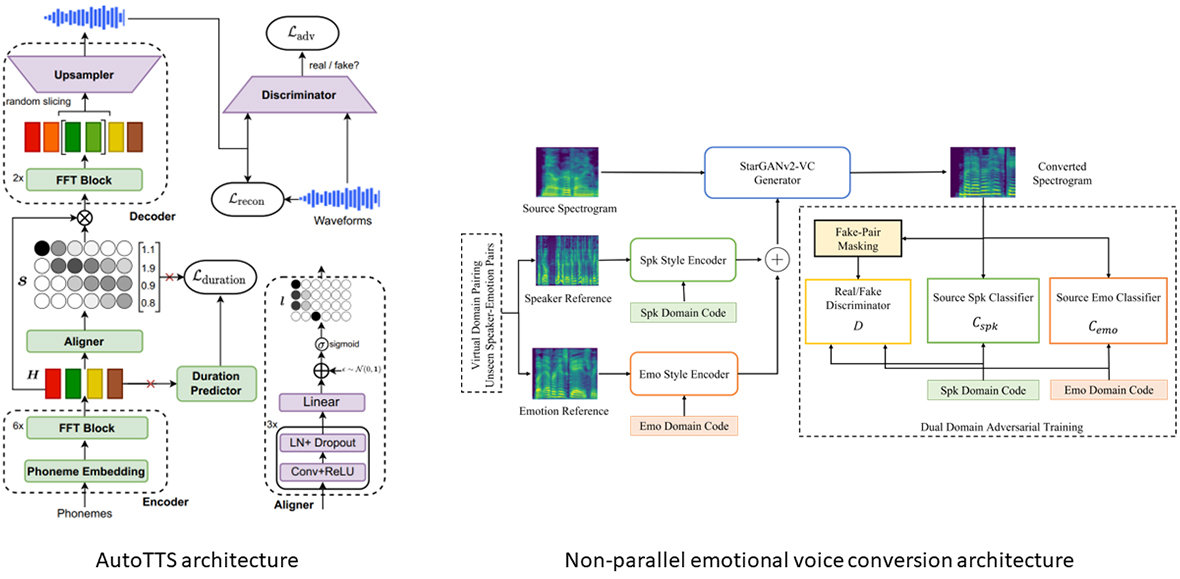
Publications
Publication 01 Hierarchical Diffusion Models for Singing Voice Neural Vocoder
- Authors
- Naoya Takahashi (Sony AI), Mayank Kumar Singh (Sony Research India), Yuki Mitsufuji (Sony AI/Sony Group Corporation)
- Abstract
- Recent progress in deep generative models has improved the quality of neural vocoders in speech domain. However, generating a high-quality singing voice remains challenging due to a wider variety of musical expressions in pitch, loudness, and pronunciations. In this work, we propose a hierarchical diffusion model for singing voice neural vocoders. The proposed method consists of multiple diffusion models operating in different sampling rates; the model at the lowest sampling rate focuses on generating accurate low-frequency components such as pitch, and other models progressively generate the waveform at higher sampling rates on the basis of the data at the lower sampling rate and acoustic features. Experimental results show that the proposed method produces high-quality singing voices for multiple singers, outperforming state-of-the-art neural vocoders with a similar range of computational costs.
Publication 02 DiffRoll: Diffusion-based Generative Music Transcription with Unsupervised Pretraining Capability
- Authors
- Kin Wai Cheuk (Sony Group Corporation Intern), Ryosuke Sawata (Sony Group Corporation), Toshimitsu Uesaka (Sony AI), Naoki Murata (Sony AI), Naoya Takahashi (Sony AI), Shusuke Takahashi (Sony Group Corporation), Dorien Herremans (Singapore University of Technology and Design), Yuki Mitsufuji (Sony AI/Sony Group Corporation)
- Abstract
- In this paper we propose a novel generative approach, DiffRoll, to tackle automatic music transcription (AMT). Instead of treating AMT as a discriminative task in which the model is trained to convert spectrograms into piano rolls, we think of it as a conditional generative task where we train our model to generate realistic looking piano rolls from pure Gaussian noise conditioned on spectrograms. This new AMT formulation enables DiffRoll to transcribe, generate and even inpaint music. Due to the classifier-free nature, DiffRoll is also able to be trained on unpaired datasets where only piano rolls are available. Our experiments show that DiffRoll outperforms its discriminative counterpart by 19 percentage points (ppt.) and our ablation studies also indicate that it outperforms similar existing methods by 4.8 ppt.
Publication 03 Unsupervised vocal dereverberation with diffusion-based generative models
- Authors
- Koichi Saito (Sony AI), Naoki Murata (Sony AI), Toshimitsu Uesaka (Sony AI), Chieh-Hsin Lai (Sony AI), Yuhta Takida (Sony AI), Takao Fukui (Sony Group Corporation), Yuki Mitsufuji (Sony AI/Sony Group Corporation)
- Abstract
- Removing reverb from reverberant music is a necessary technique to clean up audio for downstream music manipulations. Reverberation of music contains two categories, natural reverb, and artificial reverb. Artificial reverb has a wider diversity than natural reverb due to its various parameter setups and reverberation types. However, recent supervised dereverberation methods may fail because they rely on sufficiently diverse and numerous pairs of reverberant observations and retrieved data for training in order to be generalizable to unseen observations during inference. To resolve these problems, we propose an unsupervised method that can remove a general kind of artificial reverb for music without requiring pairs of data for training. The proposed method is based on diffusion models, where it initializes the unknown reverberation operator with a conventional signal processing technique and simultaneously refines the estimate with the help of diffusion models. We show through objective and perceptual evaluations that our method outperforms the current leading vocal dereverberation benchmarks.
Publication 04 Nonparallel Emotional Voice Conversion for unseen speaker-emotion pairs using dual domain adversarial network Virtual Domain Pairing
- Authors
- Nirmesh Shah (Sony Research India), Mayank Kumar Singh (Sony Research India), Naoya Takahashi (Sony AI), Naoyuki Onoe (Sony Research India)
- Abstract
- Primary goal of an emotional voice conversion (EVC) system is to convert the emotion of a given speech signal from one style to another style without modifying the linguistic content of the signal. Most of the state-of-the-art approaches convert emotions for seen speaker-emotion combinations only. In this paper, we tackle the problem of converting the emotion of speakers whose only neutral data are present during the time of training and testing (i.e., unseen speaker-emotion combinations). To this end, we extend a recently proposed StartGANv2-VC architecture by utilizing dual encoders for learning the speaker and emotion style embeddings separately along with dual domain source classifiers. For achieving the conversion to unseen speaker-emotion combinations, we propose a Virtual Domain Pairing (VDP) training strategy, which virtually incorporates the speaker-emotion pairs that are not present in the real data without compromising the min-max game of a discriminator and generator in adversarial training. We evaluate the proposed method using a Hindi emotional database.
Publication 05 An Attention-based Approach to Hierarchical Multi-label Music Instrument Classification
- Authors
- Zhi Zhong (Sony Group Corporation), Masato Hirano (Sony Group Corporation), Kazuki Shimada (Sony AI), Kazuya Tateishi (Sony Group Corporation), Shusuke Takahashi (Sony Group Corporation), Yuki Mitsufuji (Sony AI/Sony Group Corporation)
- Abstract
- Although music is typically multi-label, many works have studied hierarchical music tagging with simplified settings such as single-label data. Moreover, there lacks a framework to describe various joint training methods under the multi-label setting. In order to discuss the above topics, we introduce hierarchical multi-label music instrument classification task. The task provides a realistic setting where multi-instrument real music data is assumed. Various hierarchical methods that jointly train a DNN are summarized and explored in the context of the fusion of deep learning and conventional techniques. For the effective joint training in the multi-label setting, we propose two methods to model the connection between fine- and coarse-level tags, where one uses rule-based grouped max-pooling, the other one uses the attention mechanism obtained in a data-driven manner. Our evaluation reveals that the proposed methods have advantages over the method without joint training. In addition, the decision procedure within the proposed methods can be interpreted by visualizing attention maps or referring to fixed rules.
Publication 06 Music Mixing Style Transfer: A Contrastive Learning Approach to Disentangle Audio Effects
- Authors
- Junghyun Koo (Sony Group Corporation Intern), Marco A. Martínez-Ramírez (Sony AI), Wei-Hsiang Liao (Sony AI), Stefan Uhlich (Sony Europe), Kyogu Lee (Seoul National University), Yuki Mitsufuji (Sony AI/Sony Group Corporation)
- Abstract
- We propose an end-to-end music mixing style transfer system that converts the mixing style of an input multitrack to that of a reference song. This is achieved with an encoder pre-trained with a contrastive objective to extract only audio effects related information from a reference music recording. All our models are trained in a self-supervised manner from an already-processed wet multitrack dataset with an effective data preprocessing method that alleviates the data scarcity of obtaining unprocessed dry data. We analyze the proposed encoder for the disentanglement capability of audio effects and also validate its performance for mixing style transfer through both objective and subjective evaluations. From the results, we show the proposed system not only converts the mixing style of multitrack audio close to a reference but is also robust with mixture-wise style transfer upon using a music source separation model.
Publication 07 Streaming Joint Speech Recognition and Disfluency Detection
- Authors
- Hayato Futami (Sony Group Corporation), Emiru Tsunoo (Sony Group Corporation), Kentaro Shibata (Sony Group Corporation), Yosuke Kashiwagi (Sony Group Corporation), Takao Okuda (Sony Group Corporation), Siddhant Arora (Carnegie Mellon University), Shinji Watanabe (Carnegie Mellon University)
- Abstract
- Disfluency detection has mainly been solved in a pipeline approach, as post-processing of speech recognition. In this study, we propose Transformer-based encoder-decoder models that jointly solve speech recognition and disfluency detection, which work in a streaming manner. Compared to pipeline approaches, the joint models can leverage acoustic information that makes disfluency detection robust to recognition errors and provide non-verbal clues. Moreover, joint modeling results in low-latency and lightweight inference. We investigate two joint model variants for streaming disfluency detection: a transcript-enriched model and a multi-task model. The transcript-enriched model is trained on text with special tags indicating the starting and ending points of the disfluent part. However, it has problems with latency and standard language model adaptation, which arise from the additional disfluency tags. We propose a multi-task model to solve such problems, which has two output layers at the Transformer decoder; one for speech recognition and the other for disfluency detection. It is modeled to be conditioned on the currently recognized token with an additional token-dependency mechanism. We show that the proposed joint models outperformed a BERT-based pipeline approach in both accuracy and latency, on both the Switchboard and the corpus of spontaneous Japanese.
Publication 08 Joint Modeling of Spoken Language Understanding Tasks with Integrated Dialog History
- Authors
- Siddhant Arora (Carnegie Mellon University), Hayato Futami (Sony Group Corporation), Emiru Tsunoo (Sony Group Corporation), Brian Yan (Carnegie Mellon University), Shinji Watanabe (Carnegie Mellon University)
- Abstract
- Most human interactions occur in the form of spoken conversations where the semantic meaning of a given utterance depends on the context. Each utterance in spoken conversation can be represented by many semantic and speaker attributes, and there has been an interest in building Spoken Language Understanding (SLU) systems for automatically predicting these attributes. Recent work has shown that incorporating dialogue history can help advance SLU performance. However, separate models are used for each SLU task, leading to an increase in inference time and computation cost. Motivated by this, we aim to ask: can we jointly model all the SLU tasks while incorporating context to facilitate low-latency and lightweight inference? To answer this, we propose a novel model architecture that learns dialog context to jointly predict the intent, dialog act, speaker role, and emotion for the spoken utterance. Note that our joint prediction is based on an autoregressive model and we need to decide the prediction order of dialog attributes, which is not trivial. To mitigate the issue, we also propose an order agnostic training method. Our experiments show that our joint model achieves similar results to task-specific classifiers and can effectively integrate dialog context to further improve the SLU performance.
Publication 09 A Study on the Integration of Pipeline and E2E SLU Systems for Spoken Semantic Parsing toward STOP Quality Challenge
- Authors
- Siddhant Arora (Carnegie Mellon University), Hayato Futami (Sony Group Corporation), Shih-Lun Wu (Carnegie Mellon University), Jessica Huynh (Carnegie Mellon University), Yifan Peng (Carnegie Mellon University), Yosuke Kashiwagi (Sony Group Corporation), Emiru Tsunoo (Sony Group Corporation), Brian Yan (Carnegie Mellon University), Shinji Watanabe (Carnegie Mellon University)
- Abstract
- Recently there have been efforts to introduce new benchmark tasks for spoken language understanding (SLU), like semantic parsing. In this paper, we describe our proposed spoken semantic parsing system for the quality track (Track 1) in Spoken Language Understanding Grand Challenge which is part of ICASSP Signal Processing Grand Challenge 2023. We experiment with both end-to-end and pipeline systems for this task. Strong automatic speech recognition (ASR) models like Whisper and pretrained Language models (LM) like BART are utilized inside our SLU framework to boost performance. We also investigate the output level combination of various models to get an exact match accuracy of 80.8, which won the 1st place at the challenge.
Publication 10 E-Branchformer-based E2E SLU toward STOP On-Device Challenge
- Authors
- Yosuke Kashiwagi (Sony Group Corporation), Siddhant Arora (Carnegie Mellon University), Hayato Futami (Sony Group Corporation), Jessica Huynh (Carnegie Mellon University), Shih-Lun Wu (Carnegie Mellon University), Yifan Peng (Carnegie Mellon University), Brian Yan (Carnegie Mellon University), Emiru Tsunoo (Sony Group Corporation), Shinji Watanabe (Carnegie Mellon University)
- Abstract
- In this paper, we report our team’s study on track 2 of the Spoken Language Understanding Grand Challenge, which is a component of the ICASSP Signal Processing Grand Challenge 2023. The task is intended for on-device processing and involves estimating semantic parse labels from speech using a model with 15 million parameters. We use E2E E-Branchformer-based spoken language understanding model, which is more parameter controllable than cascade models, and reduced the parameter size through sequential distillation and tensor decomposition techniques. On the STOP dataset, we achieved an exact match accuracy of 70.9% under the tight constraint of 15 million parameters.
Publication 11 The Pipeline System of ASR and NLU with MLM-based Data Augmentation toward STOP Low-Resource Challenge
- Authors
- Hayato Futami (Sony Group Corporation), Jessica Huynh (Carnegie Mellon University), Siddhant Arora (Carnegie Mellon University), Shih-Lun Wu (Carnegie Mellon University), Yosuke Kashiwagi (Sony Group Corporation), Yifan Peng (Carnegie Mellon University), Brian Yan (Carnegie Mellon University), Emiru Tsunoo (Sony Group Corporation), Shinji Watanabe (Carnegie Mellon University)
- Abstract
- This paper describes a system for low-resource domain adaptation (Track 3) in the STOP challenge. In the track, we adopt a pipeline approach of ASR and NLU. For ASR, we finetune Whisper for each domain with upsampling. For NLU, we fine-tune BART on all the Track3 data and then on lowresource domain data. We apply masked LM (MLM) -based data augmentation, where some of input tokens and corresponding target labels are replaced using MLM. We also apply retrieval augmentation, where model input is augmented with similar training samples. As a result, we achieved exact match (EM) accuracy 63.3/75.0 (average: 69.15) for reminder/ weather domain.
Publication 12 Improving Self-Supervised Learning for Audio Representations by Feature Diversity and Decorrelation
- Authors
- Bac Nguyen (Sony Europe B.V.), Stefan Uhlich (Sony Europe B.V.), Fabien Cardinaux (Sony Europe B.V.)
- Abstract
- Self-supervised learning (SSL) has recently shown remarkable results in closing the gap between supervised and unsupervised learning. The idea is to learn robust features that are invariant to distortions of the input data. Despite its success, this idea can suffer from a collapsing issue where the network produces a constant representation. To this end, we introduce SELFIE, a novel Self-supervised Learning approach for audio representation via Feature Diversity and Decorrelation. SELFIE avoids the collapsing issue by ensuring that the representation (i) maintains a high diversity among embeddings and (ii) decorrelates the dependencies between dimensions. SELFIE is pre-trained on the large-scale AudioSet dataset and its embeddings are validated on nine audio downstream tasks, including speech, music, and sound event recognition. Experimental results show that SELFIE outperforms existing SSL methods in several tasks.
Publication 13 AutoTTS: End-to-End Text-to-Speech Synthesis through Differentiable Duration Modelingc
- Authors
- Bac Nguyen (Sony Europe B.V.), Fabien Cardinaux (Sony Europe B.V.), Stefan Uhlich (Sony Europe B.V.)
- Abstract
- Parallel text-to-speech (TTS) models have recently enabled fast and highly-natural speech synthesis. However, they typically require external alignment models, which are not necessarily optimized for the decoder as they are not jointly trained. In this paper, we propose a differentiable duration method for learning monotonic alignments between input and output sequences. Our method is based on a soft-duration mechanism that optimizes a stochastic process in expectation. Using this differentiable duration method, we introduce AutoTTS, a direct text-to-waveform speech synthesis model. AutoTTS enables high-fidelity speech synthesis through a combination of adversarial training and matching the total ground-truth duration. Experimental results show that our model obtains competitive results while enjoying a much simpler training pipeline. Audio samples are available online.
Publication 14 : Satellite Workshop, SASB 2023: Self-Supervision in Audio, Speech and Beyond Self-supervised audio encoder with contrastive pretraining for Respiratory Anomaly Detection
- Authors
- Shubham Kulkarni (Sony Group Corporation), Hideaki Watanabe (Sony Group Corporation), Fuminori Homma (Sony Group Corporation)
- Abstract
- Accurate analysis of lung sounds is essential for early disease detection and monitoring. We propose a self-supervised contrastive audio encoder for automated respiratory anomaly detection. The model consists of a direct waveform audio encoder trained in two stages. First, self-supervised pretraining using an acoustic dataset (Audioset) is used to extract high-level representations of the input audio. Second, domain-specific semi-supervised contrastive training is employed on a respiratory database to distinguish cough and breathing sounds. This direct waveform-based encoder outperforms conventional mel-frequency cepstral coefficients (MFCC) and image spectrogram features with CNN-ResNet-based detection models. It is also shown that the pretraining using varied audio sounds significantly improves detection accuracy compared to speech featurization models such as Wav2Vec2.0, PASE and HuBERT.
The proposed model achieves the highest accuracy score (91%) and inter-patient (specificity and sensitivity) evaluation score (84.1%) on the largest respiratory anomaly detection dataset. Our work further contributes to remote patient care via accurate continuous monitoring of respiratory abnormalities.
Publication 15 Towards Adversarially Robust Continual Learning
- Authors
- Tao Bai (Sony AI Intern), Chen Chen (Sony AI), Lingjuan Lyu (Sony AI), Jun Zhao, Bihan Wen
- Abstract
- Recent studies show that models trained by continual learning can achieve the comparable performances as the standard supervised learning and the learning flexibility of continual learning models enables their wide applications in the real world. Deep learning models, however, are shown to be vulnerable to adversarial attacks. Though there are many studies on the model robustness in the context of standard supervised learning, protecting continual learning from adversarial attacks has not yet been investigated. To fill in this research gap, we are the first to study adversarial robustness in continual learning and propose a novel method called Task-Aware Boundary Augmentation (TABA) to boost the robustness of continual learning models. With extensive experiments on CIFAR-10 and CIFAR-100, we show the efficacy of adversarial training and TABA in defending adversarial attacks.